Rooting Out Exceptions That Can Derail Freight Networks
Detecting and addressing service anomalies before they develop into costly disruptions is critical to efficiency
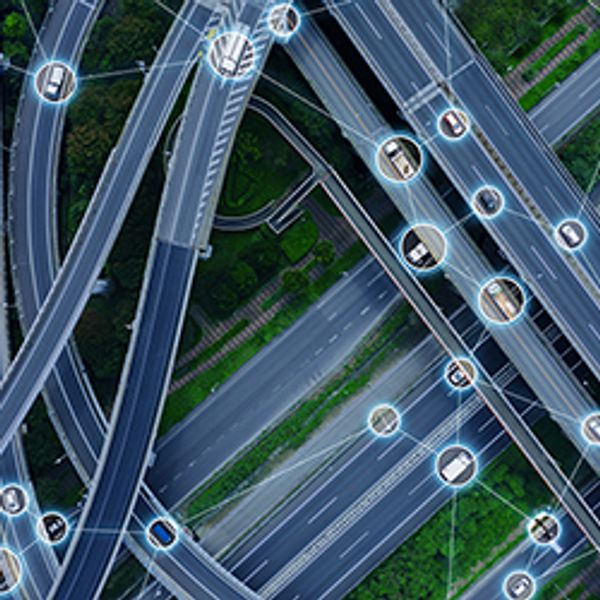
Exception management – detecting and addressing service anomalies before they develop into costly disruptions – is critical to the efficiency of freight networks. In today’s fast-paced logistics environment, there is scant room for error, and the ability to manage and eliminate these red flags is more important than ever.
Leading fourth-party logistics providers (4PL) such as Penske Logistics have long recognized this imperative. Penske uses its domain expertise to help shippers fix the problems that underlie exceptions in freight management. In addition, Penske is applying machine learning (ML) to exception management; a technology that excels at uncovering the patterns and irregularities that portend service interruptions.
Spotlighting Root Causes
To spot the inconsistencies in freight movements that can give rise to delivery failures, excellent visibility into the supply chain is a must. With the benefit of real-time or near real-time visibility into cargo flows, an experienced 4PL such as Penske Logistics can identify aberrations, assess the potential risk, and take the appropriate corrective action. Moreover, Penske keeps all authorized stakeholders informed of the actions taken, and analyzes anomalies to understand their causes and eliminate the associated risks.
For example, “we work with shippers to review supplier compliance; are they following the guidelines set by shipper customers and providing the right level of visibility into shipments,” says Tracy Urbanski, senior vice president of operations for Penske Logistics, Penske Logistics. It might be necessary to enforce the guidelines or make adjustments that provide a clearer and more timely view of the status of loads.
In some cases, Penske tweaks shipment schedules to improve supply chain visibility. Generating more granular information on the movement of loads often reveals unnoticed issues such as items that are occasionally short-shipped. When this happens, “we ask the supplier why it ships, say, 75 units rather than 100 units, and find out when it moves the balance to make up for the shortfalls,” says Urbanski. “We’ve developed a sophisticated series of tools that allows us to provide the level of visibility needed to reveal such issues.”
Unscheduled shortfalls in the number of units transported can undermine service quality in various ways. Penske uses its deep logistics expertise to pinpoint critical areas of risk.
“We’ll look at what product is on the shop floor and in other locations, what shipments are in transit, which lines are impacted, and what actions we need to take to ensure that the shipper does not suffer service disruptions,” Urbanski says. Perhaps it is necessary to expedite shipments or reconfigure the company’s inventory strategy. “We will take the required corrective action and then monitor key parameters such as inventory debits and credits while providing the shipper with all relevant scorecard information,” explains Urbanski.
The ultimate aim is to establish a robust freight schedule that consistently meets the client’s service needs.
ML Microscope
Now, Penske is deploying machine learning (ML) to purge freight operations of the exceptions that can play havoc with delivery schedules.
ML is a powerful tool that discerns patterns from large data sets and sifts through these patterns to highlight anomalies. Hence, the technology is ideally suited to the task of unearthing exceptions in complex freight networks.
“The availability of timely, accurate data from multiple sources is a key enabler of ML applications in freight transportation,” says Urbanski. In addition to tapping into internal databases, Penske layers external sources onto data sets such as updates on weather and traffic patterns and operational inputs from carriers.
As well as honing Penske’s ability to detect and fix irregularities in freight movements, ML is bringing new opportunities to manage exceptions and improve freight network performance. Examples cited by Urbanski include identifying wasteful empty mile moves and making cargo handling facilities more efficient by retuning vehicle appointment systems and reducing driver waiting times.
Untapped Potential
Looking ahead, the ability of ML to learn from experience and refine predictive models promises to deliver improvements in exception management that were unimaginable a few years ago.
“For instance, we will be able to look even further out and help shippers to anticipate future shipment arrival and departure demands and plan their labor resources to meet these demands,” says Urbanski. The discipline of managing exceptions in freight networks is evolving “as ML creates more predictability and better planning,” she adds.