Unlocking the Power of Machine Learning to Forecast Trucking Rates
In a fragmented market, shippers turn to truck pricing prediction models driven by machine learning technology
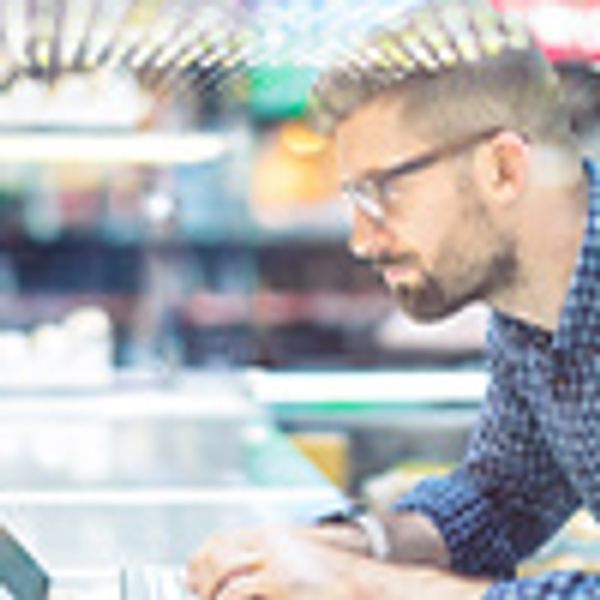
When buying truck capacity, shippers need to have a clear understanding of carrier pricing trends in relevant lanes. But in the highly fragmented U.S. truck market, freight rates vary in response to many factors, and it can be difficult to get a fix on which rates are competitive and how stable they are in specific lanes.
This is changing thanks to the arrival of truck pricing prediction models driven by machine learning (ML) technology. A similar model developed by Penske Logistics is helping shippers to understand for-hire truckload (TL) pricing movements with much greater precision. As a result, shippers are better able to negotiate competitive rates for their freight.
Freight Market Dynamics
ML is a branch of artificial intelligence (AI) that uses algorithms to identify patterns in large data sets and to learn from these patterns to predict outcomes. It's perhaps not surprising, then, that the technology's predictive capabilities are finding powerful applications in the huge, complex market for TL capacity.
"Penske operates a multibillion-dollar freight management network, and we employ ML to help forecast truckload pricing in any given lane," explains Vishwa Ram, vice president of data science & analytics at Penske Logistics.
The for-hire trucking market can be broadly divided into two sub-markets. First, the contract market pertains to long-term freight contracts shippers negotiate with individual trucking carriers. These agreements typically span a year or more. Second, there is the spot market for short-term contracts. Shippers dip into this market for various reasons. Perhaps they have exhausted their routing guides and need to find alternative carriers, or a sudden surge in demand has created an urgent need to source more carrying capacity.
The contract market tends to be the less volatile of the two. However, shippers still require a firm understanding of pricing strategies when negotiating with carriers. The spot market is more volatile, and shippers that can track pricing movements accurately are in a much better position to find capacity that is competitively priced.
Ensuring Data Integrity
Penske has devoted much effort to making its TL pricing model as accurate as possible—especially compared to other established benchmarking models based on aggregated data that give a less granular view of the market.
"We can slice and dice the data, look at certain lanes and drill down into specific freight categories to develop a clearer picture of where prices are headed," says Ram.
Using data from Penske's transactional system, rates from a wide range of carriers are fed into the model. "We frequently sweep the data in the transactional system and regenerate the model, so we can compare it to previous versions to make sure it is still accurate," Ram says.
Penske also has taken pains to make sure that the model reflects real cargo movements. In the TL world, it is common for an annual contract to break down after only a few months because the commercial priorities of one or both parties have changed. For instance, a carrier diverts capacity to other, more lucrative lanes and starts to reject loads from the original shipper it contracted with. Or a shipper's customer demands—and hence truck capacity needs—unexpectedly shift, forcing the company to reconfigure its transportation portfolio.
"Paper rates are not meaningful unless there are actual loads that match those rates. We decided to use only data based on loads that were hauled by carriers at specific rates that we know are genuine contract or spot market rates. That way we know the data in our model is solid," says Ram.
Improving Over Time
In addition to helping shippers negotiate competitive TL rates, ML-enabled prediction models inform their transportation sourcing programs. For example, the intelligence provided by the model can guide decisions on the timing of TL procurement events. Shippers also have a clearer idea of how the availability of trucks—and associated freight rates—vary by region or lane. This information helps them to decide how deep they need to go in their routing guides to source capacity at any given time, and to anticipate capacity shortages during periods of peak demand.
Penske is also using its TL model to project how trucking resources need to be allocated in a freight network for maximum efficiency. The model requires only basic inputs, such as the average number of daily stops and distance traveled, to provide a breakdown of what resources are needed to meet the network's efficiency and service goals.
Over time, ML models become more refined because they automatically learn from experience. This means that Penske's TL pricing model will become even more accurate and nuanced.
Also, Ram points out that the TL market is evolving rapidly, with the traditional truck freight brokerage businesses attempting to be disrupted by the uberization of freight services. Technologies such as ML enable lead logistics providers such as Penske to stay ahead of the competition and develop service innovations that make it easier for shippers to locate and secure TL capacity.
"Five years ago there wasn't the appetite for this kind of model. Now there is, and Penske Logistics has the technology and expertise to stay ahead of the curve," says Ram.